Data Analytics /Visualization /Tangible Interaction /Architectural /Urban Design
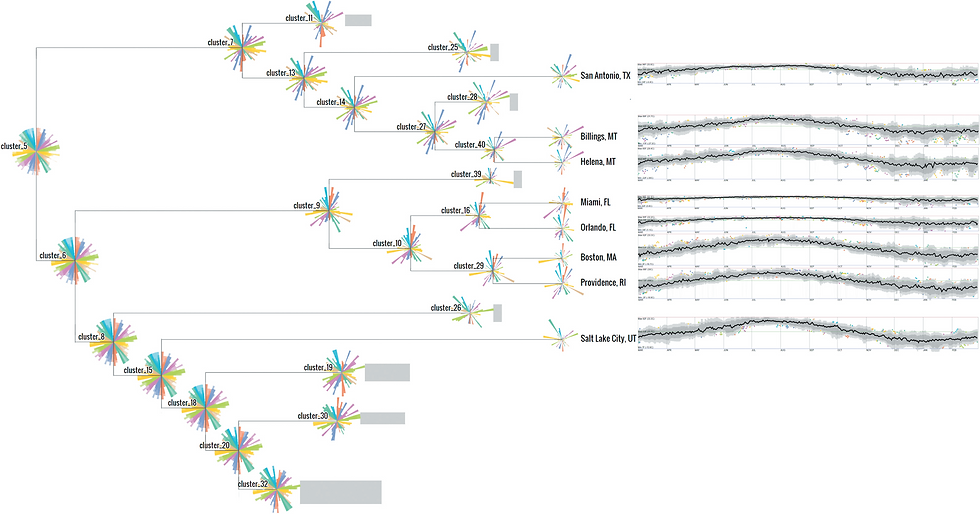
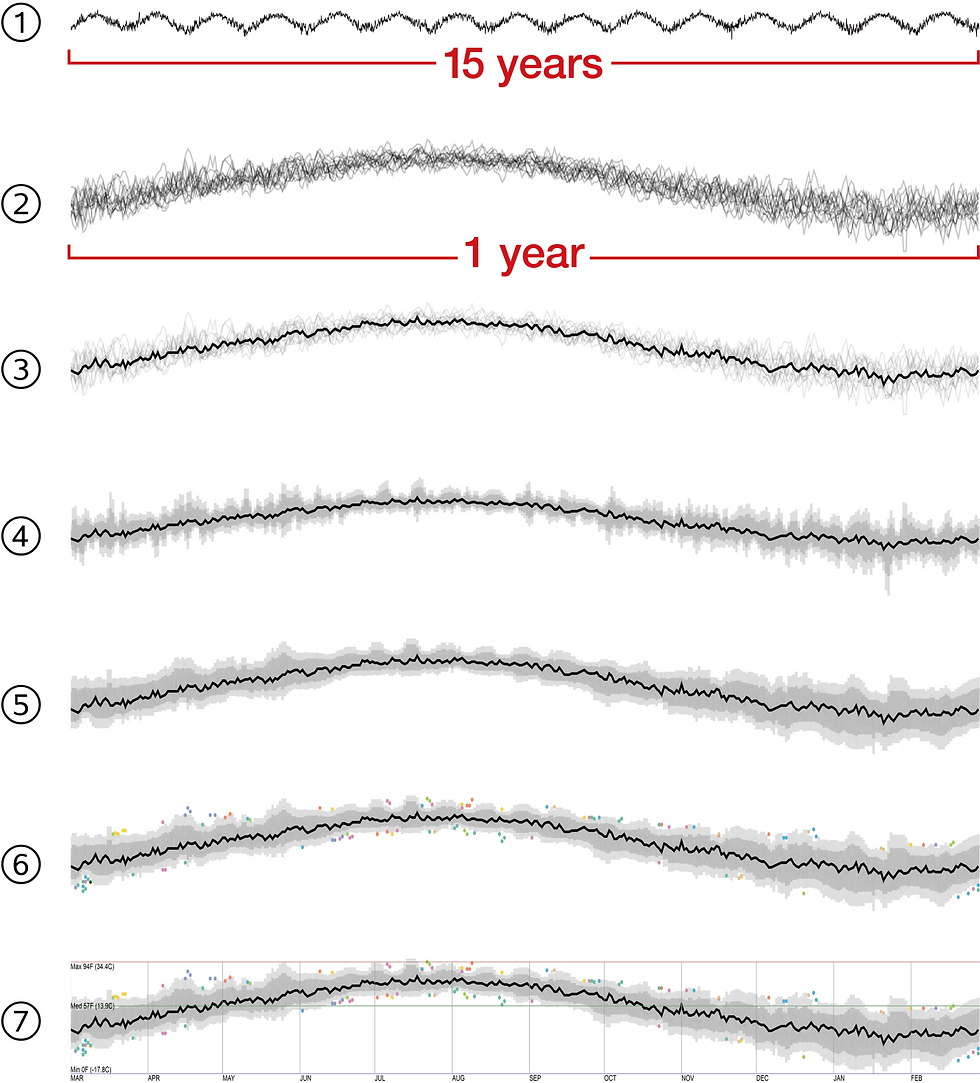

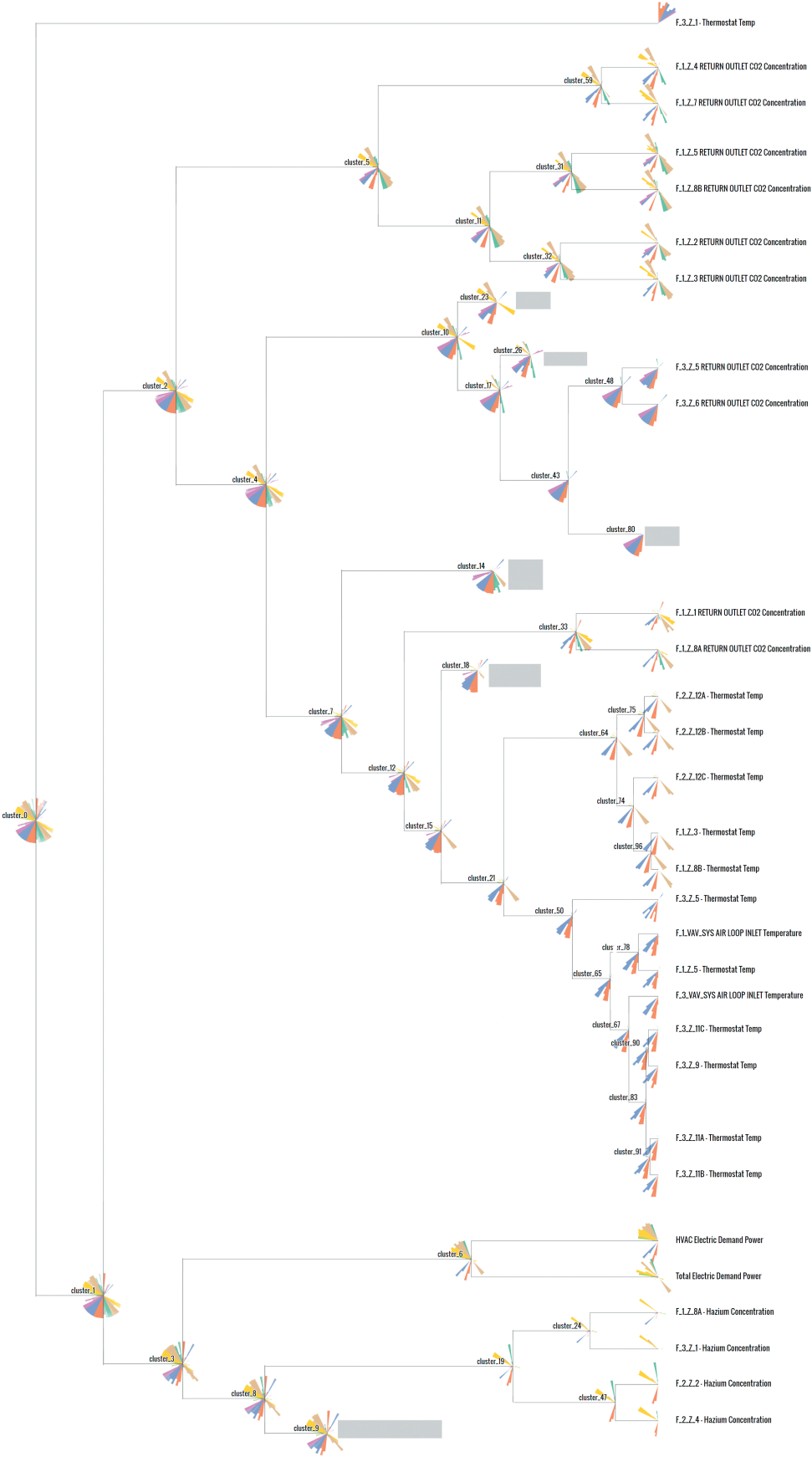
TreeRoses
2019/08
Tang, H., Shuang, W., Zhou, Z., Qian, C.Z. & Chen, Y.V. (2019). TreeRoses: Outlier-Centric Monitoring and Analysis of Periodic Time Series Data. Journal of Visualization. (pp. 1-15). doi: 10.1007/s12650-019-00586-1
Periodical time series data can reveal typical temporal patterns as well as anomalies. Although they appear in different ranges with different measurements, the variables in a dataset may be associated with and affect each other. When the quantity of datasets becomes large, monitoring and analyzing these data becomes difficult.
We propose a visual analytics framework that is capable of transforming a large number of multivariate time serial data into manageable visual representations to enable analysts to
monitor anomalies and trends. The data are sorted in two directions of temporal and categorical. We extract the temporal possibility range patterns and anomalies from each variable. Associated variables typically include similar anomalies. Therefore we use hierarchical clustering algorithm to group the variables with similar anomaly appearances.
Through our interactive visualization tool, we
find that possibility ranges, anomaly and its summary, and hierarchical association are perceptible to different degrees. We apply our
method to two periodical time series datasets to showcase how our framework effectively monitors anomalies in meteorological data
and building HVAC sensor data.
Role in the team:
Lead developer and Designer